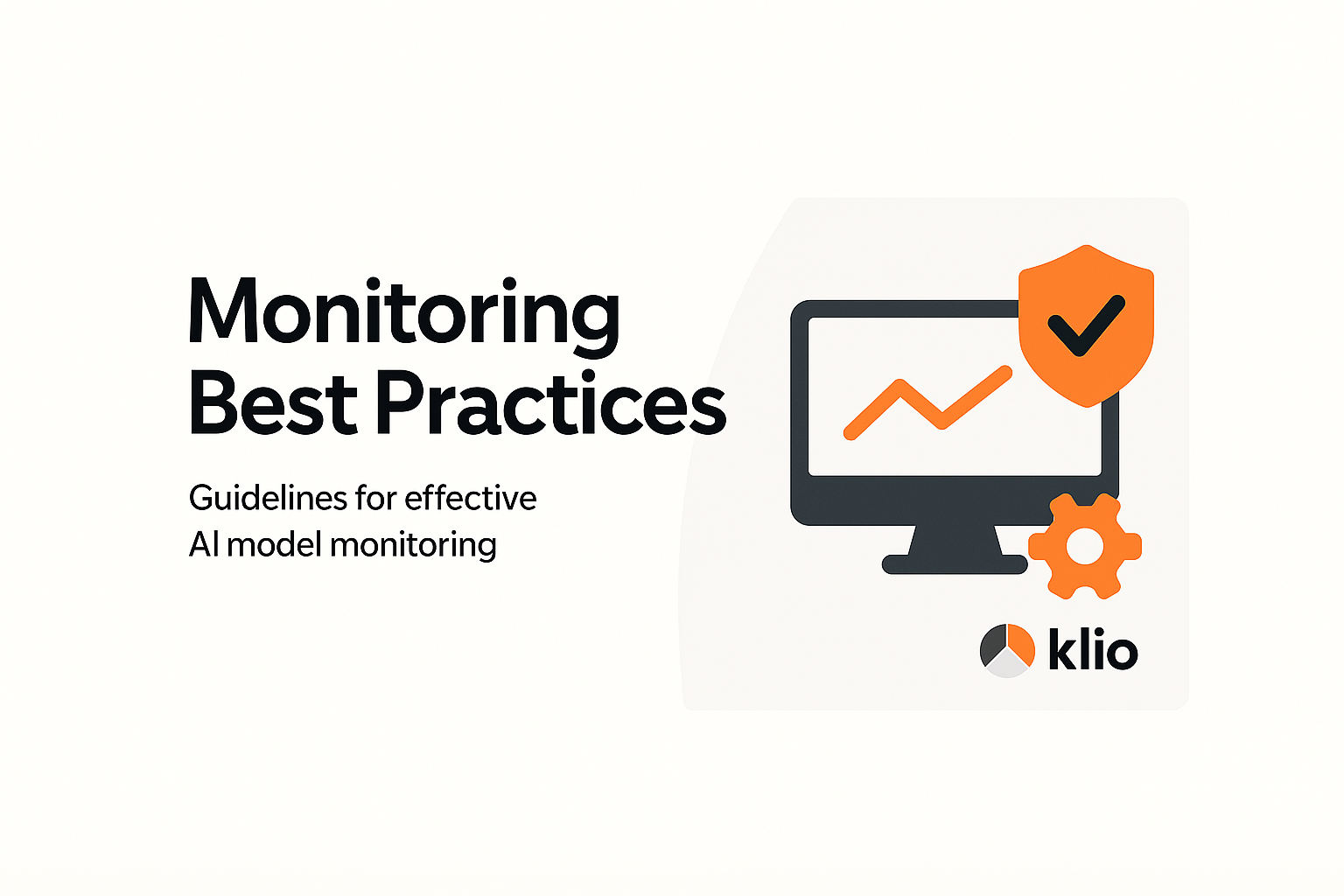
Model Monitoring Best Practices
Model Monitoring Best Practices
Deploying a machine learning model is just the beginning of its lifecycle. Without proper monitoring, models can silently degrade, leading to poor decisions and potential business impact. Here are best practices for effective model monitoring.
Why Monitor ML Models?
Machine learning models can fail in production for several reasons:
- Data drift: Changes in the distribution of input data
- Concept drift: Changes in the relationship between inputs and outputs
- System failures: Infrastructure issues affecting model performance
- Feedback loops: The model's predictions influence future input data
Key Metrics to Monitor
1. Performance Metrics
Track standard performance metrics relevant to your problem:
- Classification: accuracy, precision, recall, F1 score
- Regression: RMSE, MAE, R-squared
- Ranking: NDCG, MAP
2. Data Quality Metrics
Monitor the quality of input data:
- Missing values
- Out-of-range values
- Unexpected data types
- Feature distribution shifts
3. Operational Metrics
Track how your model operates in production:
- Prediction latency
- Throughput
- Resource utilization
- Error rates
Implementing Effective Monitoring
Set Up Automated Alerts
Define thresholds for key metrics and set up alerts when they're breached. Consider:
- Absolute thresholds based on business requirements
- Relative thresholds based on historical performance
- Statistical thresholds based on significance tests
Implement Dashboards
Create dashboards that visualize:
- Model performance over time
- Input data distributions
- Prediction distributions
- System health metrics
Establish Regular Reviews
Schedule regular reviews of model performance:
- Daily checks for critical models
- Weekly deep dives into performance trends
- Monthly comprehensive reviews with stakeholders
Advanced Monitoring Techniques
Explainability Monitoring
Track how feature importance changes over time to detect concept drift.
A/B Testing
Compare new models against baseline models in production.
Shadow Deployment
Run new models in parallel with production models to evaluate performance without affecting users.
Conclusion
Effective model monitoring is essential for maintaining ML model performance in production. By tracking the right metrics and implementing proper monitoring systems, you can detect issues early and ensure your models continue to deliver value.
The Critical Importance of Continuous Model Oversight
In the rapidly evolving world of machine learning, deploying a model is just the beginning. The real challenge lies in maintaining its performance, reliability, and effectiveness over time.
Why Model Monitoring Matters
Machine learning models are not set-it-and-forget-it solutions. They require constant vigilance due to:
- π Changing data distributions
- π Concept drift
- β οΈ Performance degradation
- βοΈ Emerging biases
Key Dimensions of Effective Model Monitoring
1. Performance Tracking
Essential Metrics to Monitor:
- π Accuracy
- π― Precision
- π Recall
- π― F1 Score
- π Mean Absolute Error
- β‘ Prediction latency
2. Data Quality Monitoring
Critical Aspects:
- π Input data distribution changes
- π Feature value drift
- β Missing or corrupted data
- π Sudden statistical shifts
3. Bias and Fairness Detection
Monitoring Priorities:
- π₯ Demographic performance disparities
- βοΈ Unintended discriminatory patterns
- π Representation imbalances
- π― Ethical AI compliance
4. Resource Utilization
Infrastructure Considerations:
- π» Computational resource consumption
- β‘ Inference time
- πΎ Memory usage
- π Scalability challenges
Practical Implementation Strategies
Continuous Validation Techniques
-
Rolling Window Analysis
- Implement rolling window evaluations
- Track temporal performance changes
-
Deployment Strategies
- Use shadow deployment
- A/B testing frameworks
-
Monitoring Infrastructure
- Create automatic alerting systems
- Develop comprehensive logging mechanisms
Advanced Monitoring Approaches
Approach | Description | Benefits |
---|---|---|
Statistical Control | Process control charts and drift detection | Early warning system |
ML Observability | Comprehensive model behavior tracking | Deep performance insights |
Automated Retraining | Self-healing model pipelines | Maintained performance |
Performance Dashboards | Real-time visualization | Quick issue identification |
Common Monitoring Challenges
Complex Model Architectures
"The more sophisticated the model, the more critical the monitoring becomes."
- π€ Increased interpretability difficulties
- π More intricate drift detection
High-Dimensional Data
- π Challenging feature tracking
- π Complex distribution analysis
Computational Overhead
- β‘ Monitoring impact on performance
- π§ Optimization requirements
Best Practices Checklist
Monitoring Framework
- Establish baseline performance metrics
- Set up automated alerting
- Create periodic performance reviews
- Implement comprehensive logging
- Design fallback mechanisms
Data Management
- Track input data distributions
- Monitor feature importance shifts
- Validate data quality consistently
- Implement data versioning
Performance Optimization
- Define clear performance thresholds
- Create automated retraining workflows
- Develop model comparison frameworks
- Establish graceful degradation protocols
The Missing Piece: Model Explainability in Monitoring
While traditional monitoring focuses on metrics and performance, there's a critical dimension that's often overlooked: model explainability. Understanding why your model makes specific decisions is just as important as tracking its performance metrics.
Why Explainability Matters in Monitoring
-
π Root Cause Analysis
- Identify the reasons behind performance degradation
- Understand feature contribution changes over time
- Detect unwanted biases in real-time
-
π Stakeholder Trust
- Provide transparent monitoring reports
- Explain performance changes to non-technical teams
- Build confidence in model decisions
-
π― Targeted Improvements
- Focus retraining efforts on problematic features
- Optimize data collection for critical variables
- Make informed model architecture decisions
Introducing Klio.dev: Unified Monitoring and Explainability
Klio.dev uniquely combines robust model monitoring with advanced explainability tools, providing a comprehensive solution for maintaining and understanding your AI systems.
Our Integrated Approach
1. Performance Monitoring with Context
- Real-time metric tracking with SHAP and LIME explanations
- Understand not just what changed, but why it changed
- Detect drift with feature-level insights
2. Explainable Alerts
- Receive alerts with accompanying explanations
- Understand the root cause of performance issues
- Make informed decisions quickly
3. Actionable Insights
- Transform monitoring data into clear recommendations
- Prioritize improvements based on feature importance
- Track the impact of model updates with explanation trails
The Klio.dev Advantage
Feature | Traditional Monitoring | Klio.dev's Approach |
---|---|---|
Performance Tracking | β Metric-based only | β Metrics + Explanations |
Drift Detection | β Statistical tests | β Feature-level understanding |
Alert System | β Threshold-based | β Context-aware with explanations |
Root Cause Analysis | β Manual investigation | β Automated with SHAP/LIME |
Stakeholder Reports | β Technical metrics | β Clear, explainable insights |
The Future of Model Oversight
The future of ML monitoring isn't just about tracking numbersβit's about understanding the story behind them. By combining robust monitoring with powerful explainability tools, Klio.dev empowers teams to:
- π― Proactively identify and understand issues
- π‘ Make informed decisions based on clear insights
- π€ Build trust with stakeholders through transparency
- π Continuously improve model performance with context